Data reclassification¶
Reclassifying data based on specific criteria is a common task when doing GIS analysis. The purpose of this lesson is to see how we can reclassify values based on some criteria which can be whatever, such as:
1. if available space in a pub is less than the space in my wardrobe
AND
2. the temperature outside is warmer than my beer
------------------------------------------------------
IF TRUE: ==> I go and drink my beer outside
IF NOT TRUE: ==> I go and enjoy my beer inside at a table
Even though, the above would be an interesting study case, we will use slightly more traditional cases to learn classifications. We will use Corine land cover layer from year 2012, and a Population Matrix data from Estonia to classify some features of them based on our own self-made classifier, or using a ready made classifiers that are commonly used e.g. when doing visualizations.
The target in this part of the lesson is to:
- classify the bogs into big and small bogs where
- a big bog is a bog that is larger than the average size of all bogs in our study region
- a small bog ^ vice versa
- use ready made classifiers from pysal -module to classify municipal into multiple classes.
Download data¶
Download (and then extract) the dataset zip-package used during this lesson from this link.
You should have following Shapefiles in the data
folder:
corine_legend/ corine_tartu.shp population_admin_units.prj
corine_tartu.cpg corine_tartu.shp.xml population_admin_units.sbn
corine_tartu.dbf corine_tartu.shx population_admin_units.sbx
corine_tartu.prj L4.zip population_admin_units.shp
corine_tartu.sbn population_admin_units.cpg population_admin_units.shp.xml
corine_tartu.sbx population_admin_units.dbf population_admin_units.shx
Data preparation¶
Before doing any classification, we need to prepare our data a little bit.
Let’s read the data in and have a look at the columnsand plot our data so that we can see how it looks like on a map.
import pandas as pd
import geopandas as gpd
import matplotlib.pyplot as plt
# File path
fp = r"Data\corine_tartu.shp"
data = gpd.read_file(fp)
Let’s see what we have.
In [1]: data.head(5)
Out[1]:
code_12 ... geometry
0 111 ... POLYGON Z ((5290566.200000002 4034511.45 0, 52...
1 112 ... (POLYGON Z ((5259932.400000001 3993825.37 0, 5...
2 112 ... POLYGON Z ((5268691.710000002 3996582.90000000...
3 112 ... POLYGON Z ((5270008.730000001 4001777.44000000...
4 112 ... POLYGON Z ((5278194.410000001 4003869.23000000...
[5 rows x 7 columns]
We see that the Land Use in column “code_12” is numerical and we don’t know right now what that means. So we should at first join the “clc_legend” in order to know what the codes mean:
import pandas as pd
fp_clc = r"Data\corine_legend\clc_legend.csv"
data_legend = pd.read_csv(fp_clc, sep=';', encoding='latin1')
data_legend.head(5)
We could now try to merge / join the two dataframes, ideally by the ‘code_12’ column of “data” and the “CLC_CODE” of “data_legend”.
display(data.dtypes)
display(data_legend.dtypes)
# please don't actually do it right now, it might cause extra troubles later
# data = data.merge(data_legend, how='inner', left_on='code_12', right_on='CLC_CODE')
But if we try, we will receive an error telling us that the columns are of different data type and therefore can’t be used as join-index. So we have to add a column where have the codes in the same type. I am choosing to add a column on “data”, where we transform the String/Text based “code_12” into an integer number.
In [2]: def change_type(row):
...: code_as_int = int(row['code_12'])
...: return code_as_int
...:
In [3]: data['clc_code_int'] = data.apply(change_type, axis=1)
In [4]: data.head(2)
Out[4]:
code_12 ... clc_code_int
0 111 ... 111
1 112 ... 112
[2 rows x 8 columns]
Here we are “casting” the String-based value, which happens to be a number, to be interpreted as an actula numeric data type.
Using the int()
function. Pandas (and therefore also Geopandas) also provides an in-built function that provides similar functionality astype() ,
e.g. like so data['code_astype_int'] = data['code_12'].astype('int64', copy=True)
Both versions can go wrong if the String cannot be interpreted as a number, and we should be more defensive (more details later, don’t worry right now).
Now we can merge/join the legend dateframe into our corine landuse dataframe:
In [5]: data = data.merge(data_legend, how='inner', left_on='clc_code_int', right_on='CLC_CODE', suffixes=('', '_legend'))
We have now also added more columns. Let’s drop a few, so we can focus on the data we need.
In [6]: selected_cols = ['ID','Remark','Shape_Area','CLC_CODE','LABEL3','RGB','geometry']
# Select data
In [7]: data = data[selected_cols]
# What are the columns now?
In [8]: data.columns
Out[8]: Index(['ID', 'Remark', 'Shape_Area', 'CLC_CODE', 'LABEL3', 'RGB', 'geometry'], dtype='object')
Before we plot, let’s check the coordinate system.
# Check coordinate system information
In [9]: data.crs
Out[9]:
{'ellps': 'GRS80',
'lat_0': 52,
'lon_0': 10,
'no_defs': True,
'proj': 'laea',
'units': 'm',
'x_0': 4321000,
'y_0': 3210000}
Okey we can see that the units are in meters, but …
… geographers will realise that the Corine dataset is in the ETRS89 / LAEA Europe coordinate system, aka EPSG:3035. Because it is a European dataset it is in the recommended CRS for Europe-wide data. It is a single CRS for all of Europe and predominantly used for statistical mapping at all scales and other purposes where true area representation is required.
However, being in Estonia and only using an Estonian part of the data, we should consider reprojecting it into the Estonian national grid (aka Estonian Coordinate System of 1997 -> EPSG:3301) before we plot or calculate the area of our bogs.
In [10]: data_proj = data.to_crs(epsg=3301)
# Calculate the area of bogs
In [11]: data_proj['area'] = data_proj.area
# What do we have?
In [12]: data_proj['area'].head(2)
Out[12]:
0 514565.037797
1 153.104211
Name: area, dtype: float64
Let’s plot the data and use column ‘CLC_CODE’ as our color.
In [13]: data_proj.plot(column='CLC_CODE', linewidth=0.05)
Out[13]: <matplotlib.axes._subplots.AxesSubplot at 0x150118b5dd8>
# Use tight layout and remove empty whitespace around our map
In [14]: plt.tight_layout()

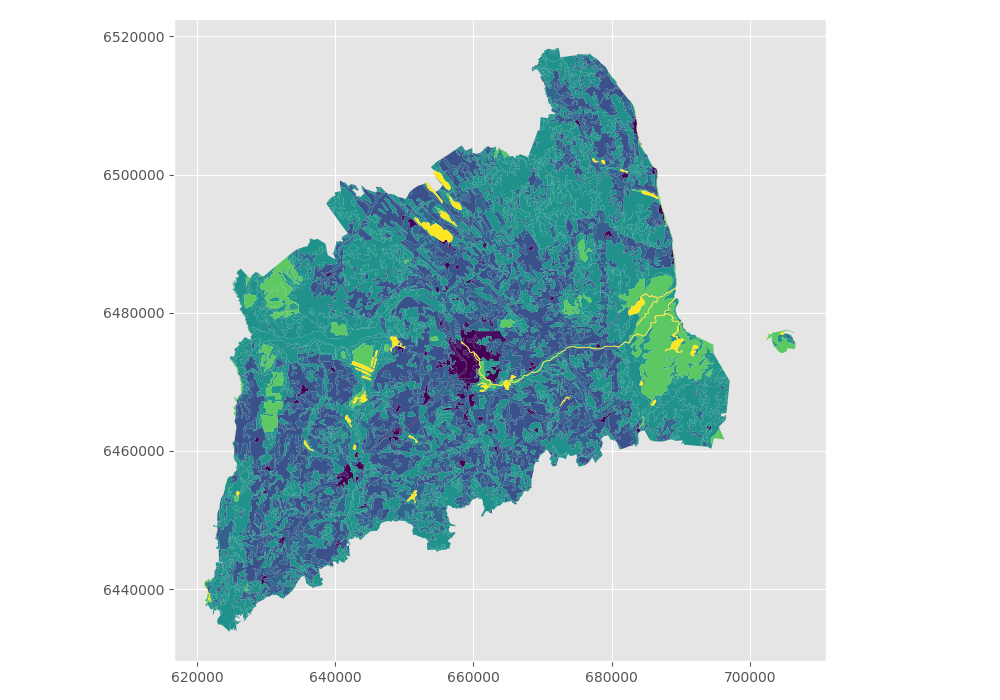
Let’s see what kind of values we have in ‘code_12’ column.
In [15]: print(list(data_proj['CLC_CODE'].unique()))
[111, 112, 121, 122, 124, 131, 133, 141, 142, 211, 222, 231, 242, 243, 311, 312, 313, 321, 324, 411, 412, 511, 512]
In [16]: print(list(data_proj['LABEL3'].unique()))